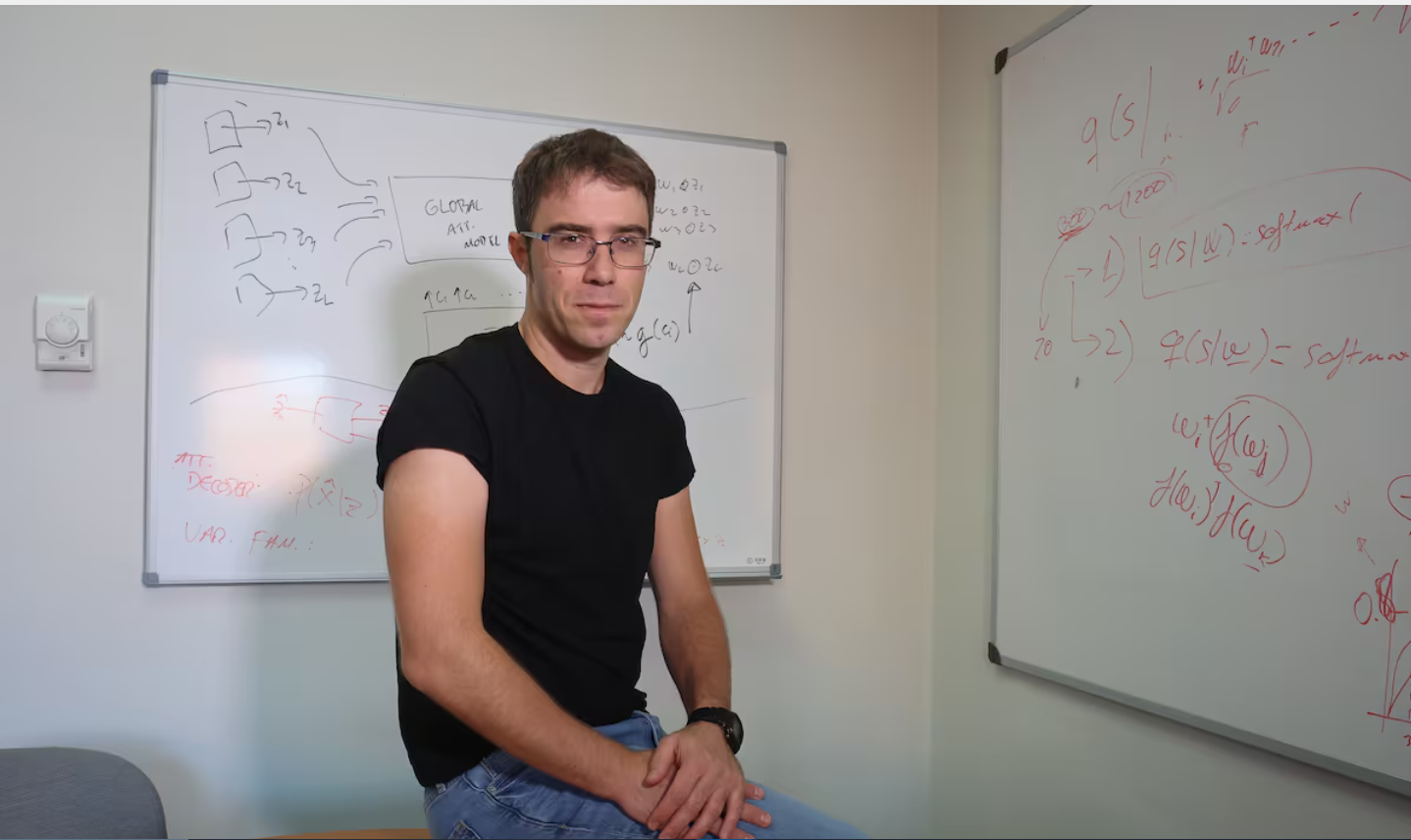
Pablo Martínez Olmos says that current algorithms that power generative tools, such as ChatGPT, are imprecise and easy to fool.
Artificial intelligence is guilty of arrogance. It is not that it is doing it on purpose, because it does not think or reason, but the algorithms that power tools such as ChatGPT or DALL-E are calibrated in such a way that, when a request is made or a question is posed to them, they end up giving very detailed answers even if they do not have a clear basis for generating them.
“Overconfidence ” is the diagnosis of Pablo Martínez Olmos (Granada, 40 years old), a doctor in Telecommunications Engineering who proposes the development of a “humble” artificial intelligence, capable of declaring the level of uncertainty about the results it gives.
“I liked the idea of humility because we think that a person with this virtue is someone who is aware of their limitations and holds back when it comes to responding when they have no idea about a topic,” says Martínez, sitting in his office in the Department of Signal Theory and Communications at the Carlos III University of Madrid.
The engineer has just been awarded one of the Leonardo Scholarships that the BBVA Foundation awards to promote “innovative projects” in the areas of science and culture. Humility is, therefore, knowing how not to give a very detailed or elaborate solution when you really don’t know the answer. Something that artificial intelligence is failing at.
It is enough to ask DALL-E —OpenIA ’s artificial intelligence program that creates images from textual descriptions— to generate a detailed map of Spain to make this bias evident. Martínez has tried it. “The shape of the country is well captured, but then the map includes very detailed aspects of the geography, such as mountains, rivers or names of regions, which are totally false,” he points out.
Then, he adds: “The algorithms are trained with certain design biases that lead them to commit what we call hallucinations , very detailed outputs that have no real basis.” These hallucinations mean that, when asked the same question, in this case, a map of Spain, the tool generates very different results. “In isolation, each map seems to make sense, because they are constructed in a very fine way, but if you look closely, they are riddled with errors.”
This brings with it several problems. One of them is the lack of rigor in the solutions offered by AI.
Another is that generative tools are more exposed to malicious use. The algorithms on these platforms have several control mechanisms to limit some responses that can give potentially dangerous or private information, such as instructions for making a homemade explosive.
However, Martínez explains that “the heart of the algorithm remains unreliable when it comes to hallucinations and, therefore, is very confident in the level of detail of its responses.” It works the same as with people: the more self-confident an algorithm is, the easier it will be to find its “tickles and fool it.”
If you ask it directly, it won’t tell you, but, with a little skill, ChatGPT can end up explaining how to cook methamphetamines at home.
Rethinking algorithms
Martínez points out that his line of research towards humble artificial intelligence is based on thinking about how to reformulate algorithms to restrict their ability to provide arbitrarily detailed solutions that are not based on reliable information.
The ultimate goal is to make it more useful, reliable and secure. Achieving this involves several computational challenges. “One of the ideas we propose is to make life more complicated for the neural network during its training, using malicious and contradictory attacks,” he points out.
It is also important to increase the restrictions that the algorithm has to store all the information on which it bases its responses so that there is “a certain order.”
Martínez uses the example of a small child’s toy room. There, Lego pieces, action figures, paints and decks of cards coexist chaotically. At first glance, everything is scattered around the room without any particular criteria, but the child, with a great ability to remember details, knows perfectly where everything is, so when he wants to put together a particular puzzle, he will know where to go to look for the pieces.
Only also, because of the disorder, he will use parts of other games that, on this occasion, will not make sense for the puzzle he wants to put together. However, if each game were kept in a specific drawer or case, it would be less likely that elements from other games would sneak in.
The same thing happens with the information stored by the algorithms; a little order is needed to reduce the margin of error. Just as it is difficult to educate a child to maintain this structure, something similar happens with the neural network of a generative artificial intelligence in computational and mathematical terms.
The engineer knows that he cannot compete with the development of large technology companies, but his project attempts to propose a more robust algorithm training methodology that will lead to the development of more reliable tools. However, no solution is magic.
“The word ‘intelligence’ in all this is very confusing. I prefer to talk about mathematical functions that we are interpolating and that, for now, cannot do without the critical capacity or supervision of the human being,” he says.
This idea of a humble, safe, traceable and controlled artificial intelligence would have, Martínez believes, a concrete application in the medical field . Detecting unknown biomarkers, designing personalized therapeutic effects, reducing the negative consequences of certain treatments and improving people’s quality of life are some of the potential uses that the design of algorithms could have if they were a little more humble.