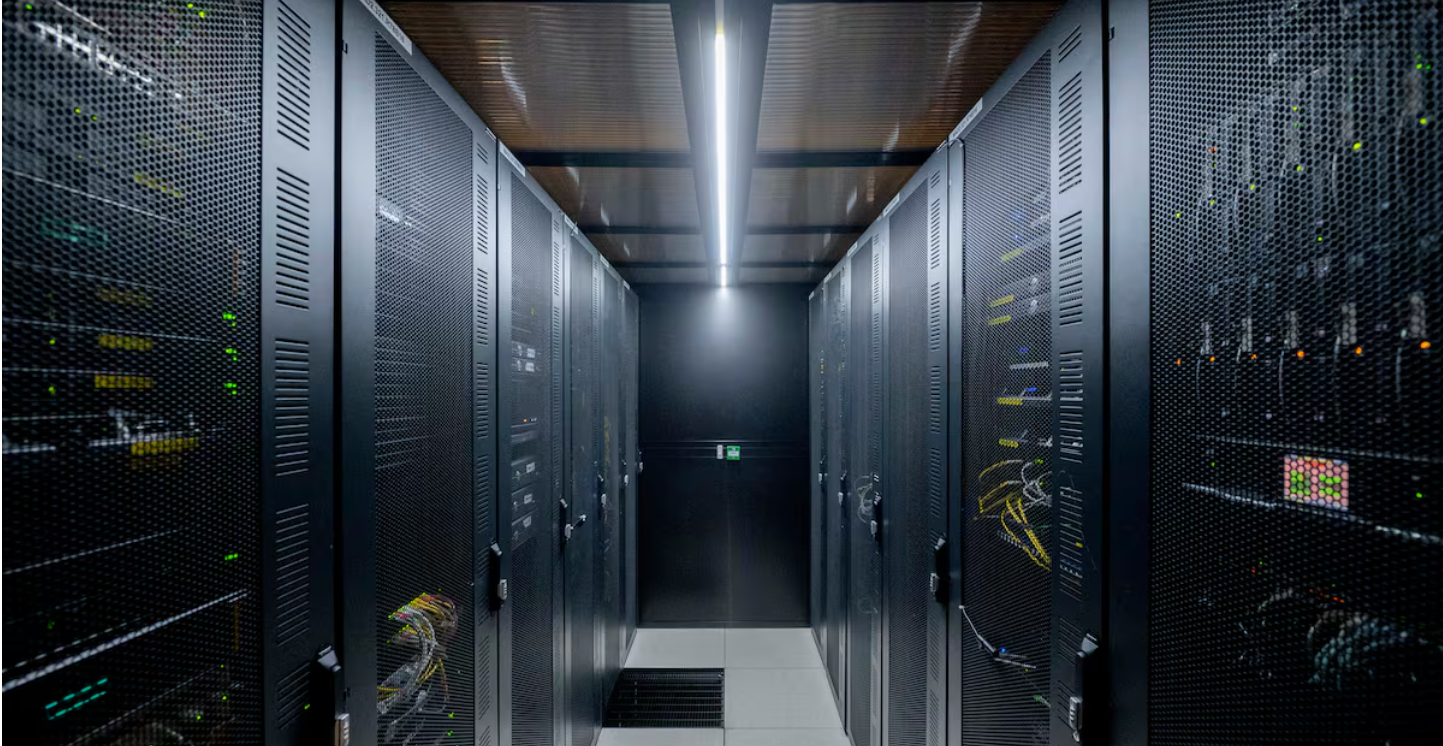
A study estimates that if waste reduction measures are not taken, obsolete equipment in data centers by 2030 will cause a major environmental problem.
Tech giants like Google, Microsoft, and Meta are engaged in a frenetic race to develop more and more tools based on generative artificial intelligence (AI). This competition is leaving its mark on the planet. Training and maintaining these sophisticated models requires enormous computing power running 24 hours a day in data centers . This has increased the energy consumption of these infrastructures , as well as their associated carbon emissions and the use of water, used to cool the systems . But the environmental footprint of generative AI does not end here.
The equipment used in the data centers where this technology is cooked must be continuously renewed, and this produces a large amount of digital waste, including highly toxic metals, such as lead or chromium. A group of scientists has made calculations and their conclusion is alarming: if measures are not taken to reduce the electronic waste associated with generative AI, it will multiply by 1,000 by 2030, reaching between 1.2 and 5 million tons.
The research, whose results are published today in the journal Nature Computational Science , aims to contribute to finding a way to reduce the electronic waste associated with generative AI, in particular with large language models (LLM) such as ChatGPT, Copilot, Gemini, Claude or Llama. “I am quite optimistic about the possibility of promoting circular economy strategies among the main actors involved in generative AI,” Peng Wang, a researcher at the Institute of Urban Environment of the Chinese Academy of Sciences in Xiamen and one of the authors of the study, told this newspaper.
“However, I am deeply concerned about the competition between the rate of expansion of generative AI and the adoption of the circular economy. Given the unprecedented increase in demand for this technology, to win this battle, shock measures should be implemented imminently,” he adds.
Wang and his colleagues used the eight-unit Nvidia DGX H100 GPU server from 2023 as a reference for their calculations. This is currently the reference hardware for AI processing, which requires much more powerful equipment than that used in other programs that run online. The authors of the study have built four future scenarios to estimate the evolution of generative AI, and its associated demand for data processing, between 2020 and 2030.
The first considers a limited expansion of the chip industry and generative AI-related manufacturing. It places growth at 41%, the same as that experienced between 2022 and 2023, understanding that it is impossible for the pace to be slower because since then improved versions of several of the most used models have been announced or presented.
The next three scenarios are the conservative one (+85%), which is based on the growth figures achieved by Alexa-type voice assistants; the moderate one (+115%), inspired by TikTok’s numbers; and the aggressive one (+136%). For the latter, it has been considered that large language models become “a ubiquitous tool in people’s daily lives”, taking as a reference the growth rate of Facebook, “a platform used literally by everyone”.
According to their calculations, waste generation would increase from 2,600 tonnes in 2023 to between 0.4 and 2.5 million tonnes by 2030 – up to 1,000 times higher. That volume of waste would be equivalent to throwing away between 2.1 and 13.3 billion iPhone 15 Pros. Or, in other words, between 0.2 and 1.6 phones per human being that year. The estimate assumes that no measures to reduce digital waste will be implemented during the current decade. In comparative terms, all waste in 2022 related to information technology equipment, such as laptops or tablets, was 4.6 million tonnes. It is estimated that by 2030 it could be 43.2 million tonnes.
Peng and his colleagues’ projections have not taken into account something that they point out could make the situation worse: restrictions on the import of semiconductors, considered key products from a geostrategic point of view. This blockage could mean that many countries will not benefit from the continued improvements in chip efficiency.
And that has its weight: the researchers’ analysis concludes that a year of delay in obtaining cutting-edge semiconductors can lead to a 14% increase in the generation of generative AI servers that reach the end of their useful life. In absolute terms, that year of delay would mean an additional 5.7 million tons of waste. Since data centers dedicated to AI are quite geographically concentrated, digital waste will be concentrated in Europe (14%), East Asia (25%) and North America (58%), although it ends up being mostly shipped to Africa and Asia.
Are the projections drawn up by Wang and his colleagues reasonable? “I think so, but I get the impression that the accumulation of decisions that simplify the model (which are necessary, because otherwise the analysis would not be viable) add a lot of uncertainty to the results and conclusions. Therefore, it is important to take these results with a grain of salt,” says Alex Hernandez, a principal investigator at the Quebec Institute for Artificial Intelligence (MILA), an institution that includes Yoshua Bengio, considered one of the godfathers of neural networks .
Hernandez also thinks that the fact that the four scenarios presented do not include an analysis of their probability, or at least of their viability, makes the study less criticizable, but also less relevant.
“Although predicting future hardware development is difficult, I believe the document’s forecast is a reasonable indicator of the e-waste that generative AI is likely to generate,” said Shaolei Ren, associate professor of electrical engineering and computer science at the University of California, Riverside (United States), in statements to the SMC Spain service.
To do
Faced with this situation, the authors examine various circular economy strategies to try to limit waste generation. The most effective, logically, is to increase the useful life of the hardware. The team of scientists’ numbers reveal that 62% of the AI servers that are thrown away each year (3.1 million tons) could be maintained if their useful life was increased by one year, which, according to the authors, is usually three.
“According to my own research, GPUs have a life cycle of between three and five years. That is, data centers dedicated to AI renew all their chips every four years or sooner,” says Ana Valdivia, professor of Artificial Intelligence, Government and Policies at the Internet Institute of the University of Oxford. The engineer, who has not participated in this study, researches precisely electronic waste.
Reusing some parts of GPU processors (those used in training AI models), such as communication, memory or battery modules, could reduce electronic waste by 42% (2.1 million tons). Valdivia does not see this as being feasible. “GPUs cannot be inserted into a circular economy because it is very expensive to recycle their components, something that I do not see discussed in the article [by Wang and his colleagues]. 100% of a GPU ends up incinerated or in a landfill,” says the expert.
The equipment used in data centers consists of three main components, according to the study: semiconductor circuits, batteries and structural parts. When discarded, toxic materials are left behind, from lead and chromium to acrylonitrile and polycarbonates, but also precious metals such as gold, silver, platinum, nickel and palladium. If properly recycled, these materials could be worth between $14 billion and $28 billion, Wang and his team estimate.
However, the study could have been more specific. “I am surprised that server cooling devices are excluded from the analysis, when they play a fundamental role in this type of device and also represent a large amount of the physical material of the servers,” says Hernández.